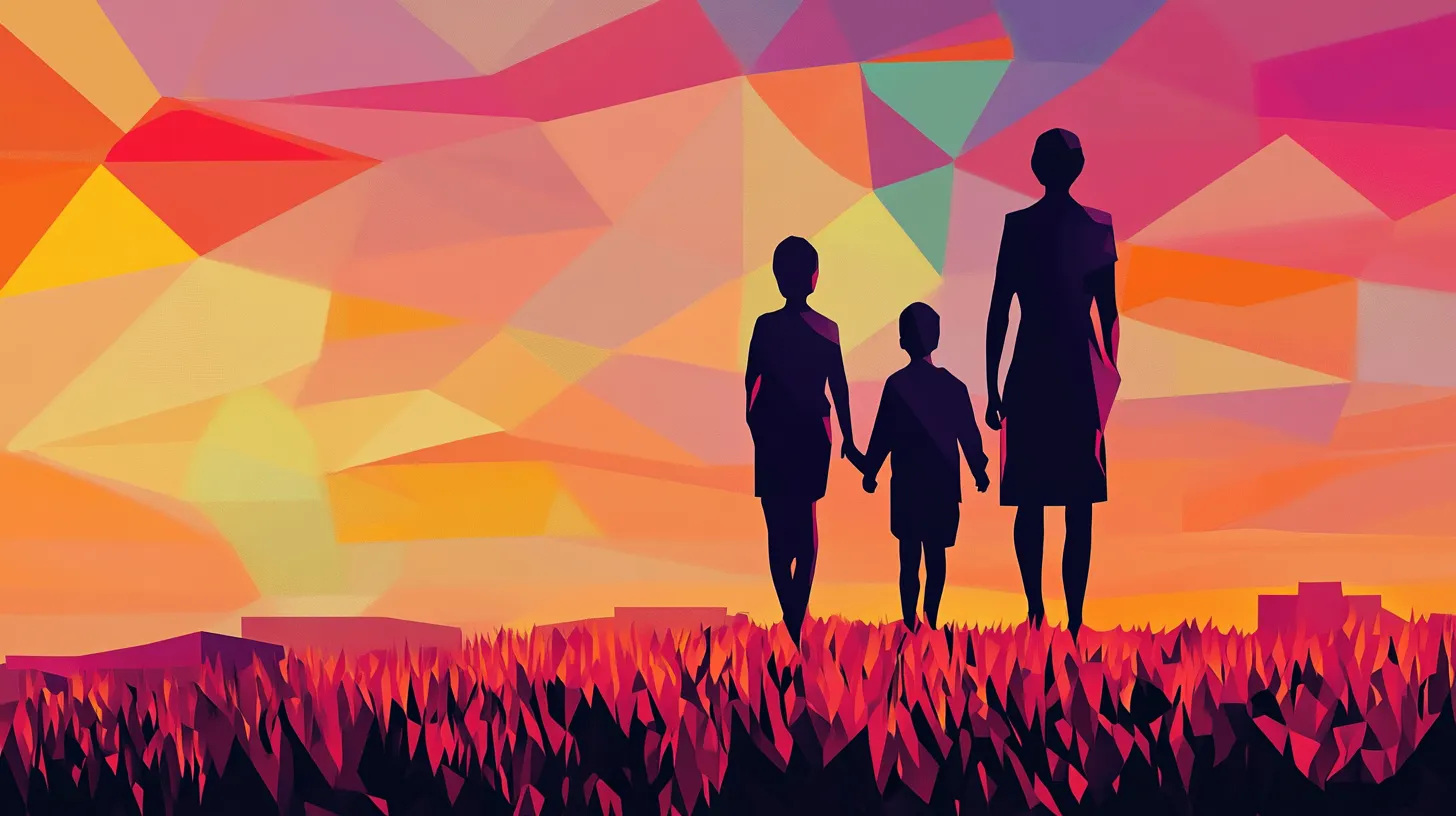
Investigating the Impact of Artificial Intelligence on Child Welfare Decision-Making
University of Georgia assistant professor Daniel Gibbs has conducted a four-year study examining the integration of artificial intelligence (AI) in child welfare systems. His research, published in the Journal of Technology in Human Services, evaluates the effectiveness and implications of AI-driven decision-making tools in child welfare investigations.
Introduction:
The adoption of AI in child welfare aims to enhance decision-making processes by analyzing extensive data to predict risks and outcomes. Gibbs’ study focuses on an AI tool used in two urban counties in a western state, designed to assess the likelihood of a child entering foster care within two years. This tool assigns a risk score between 1 and 20, guiding social workers in their evaluations.
Key Findings from the Study
Gibbs’ research highlights several critical insights:
- Data Bias Concerns: The AI tool relies on data from public systems, which may not represent the full spectrum of experiences, potentially reinforcing existing biases.
- Human-AI Interaction: The effectiveness of AI tools is significantly influenced by how social workers interpret and utilize the generated risk scores.
- Need for Improved Data: Enhancing the quality and inclusivity of data is essential to mitigate biases and improve AI tool accuracy.
Implications for Child Welfare Practice
- Balancing AI and Human Judgment: While AI can provide valuable insights, it should complement, not replace, the professional judgment of social workers.
- Addressing Ethical Concerns: The potential for data bias necessitates ethical considerations to prevent reinforcing systemic inequalities.
- Continuous Evaluation: Ongoing assessment of AI tools is crucial to ensure they adapt to evolving data and effectively support child welfare objectives.
Frequently Asked Questions:
Q1: What is the primary purpose of integrating AI into child welfare?
A1: AI aims to enhance decision-making by analyzing data to predict risks, thereby supporting social workers in identifying children who may need intervention.
Q2: What are the concerns associated with using AI in this field?
A2: Key concerns include data bias, ethical implications, and the potential over-reliance on AI at the expense of human judgment.
Q3: How can the effectiveness of AI tools in child welfare be improved?
A3: Improving data quality, ensuring ethical use, and integrating AI insights with professional expertise can enhance effectiveness.
Conclusion:
Daniel Gibbs’ study underscores the potential of AI to support child welfare decisions while highlighting the need for careful implementation to address biases and ethical concerns. As AI continues to evolve, its role in child welfare will depend on balancing technological advancements with human expertise to ensure equitable and effective outcomes. For a detailed exploration of this study, visit The Imprint.